Abstract
Millimeter-wave (mmWave) communication systems rely on narrow beams to achieve sufficient receive signal power. Adjusting these beams is typically associated with large training overhead, which becomes particularly critical for highly-mobile applications. Beam selection can benefit from the knowledge of user positions to reduce the overhead in mmWave beam training. Prior work, however, studied this problem using only synthetic data that does not accurately represent real-world measurements. In this paper, we revisit the position-aided beam prediction problem in light of real-world measurements with commercialoff-the-shelf GPS to derive insights into how much beam training overhead can be saved in practice. We also compare algorithms that perform well in synthetic data but fail to generalize with real data, and attempt to answer what factors cause inference degradation. Further, we propose a machine learning evaluation metric that better captures the end communication system objective. This work aims at closing the gap between reality and simulations in position-aided beam alignment
Video
Figures
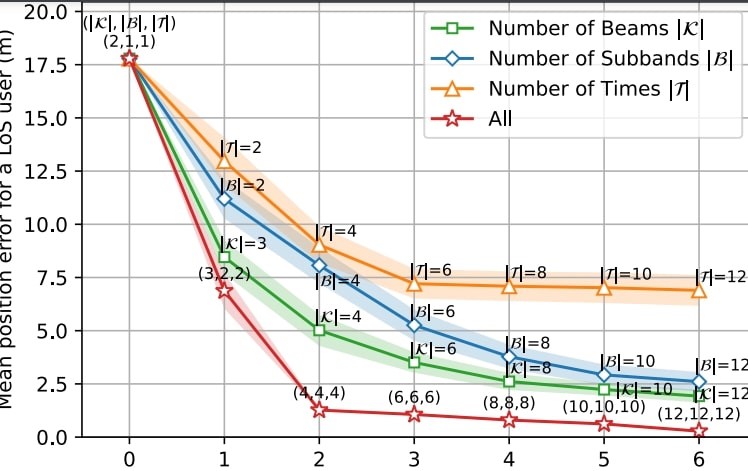
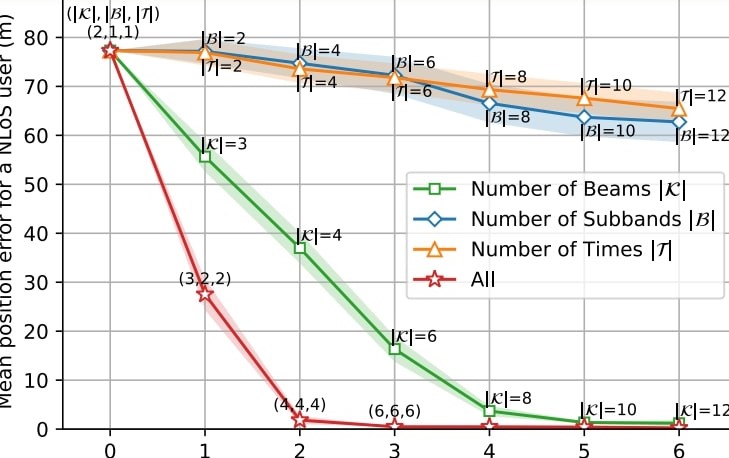
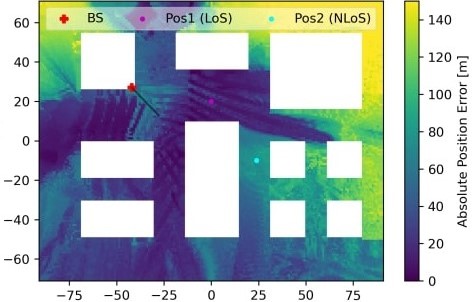
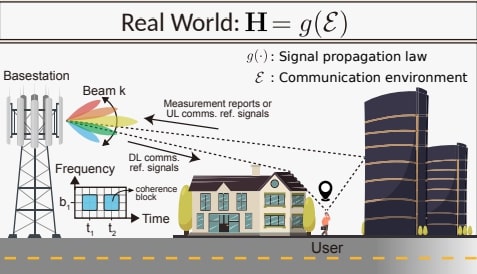
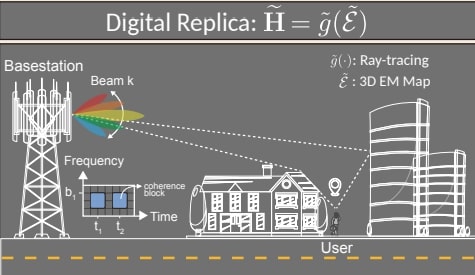
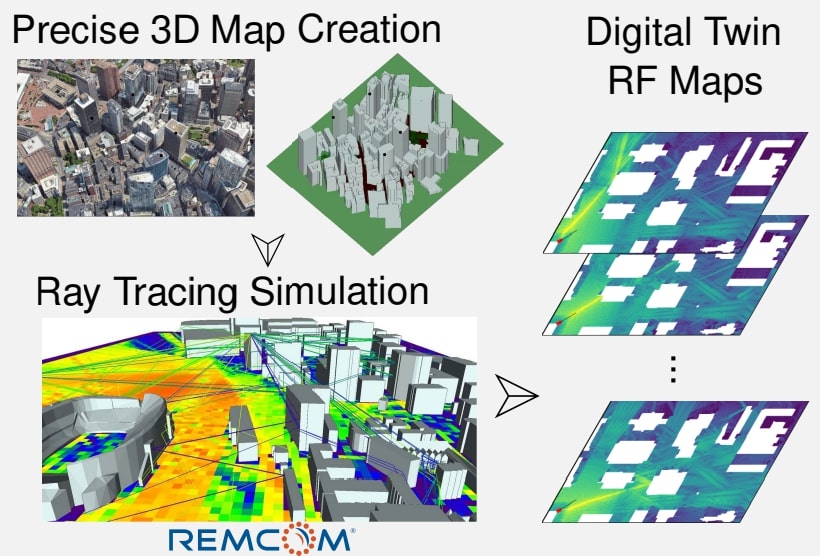
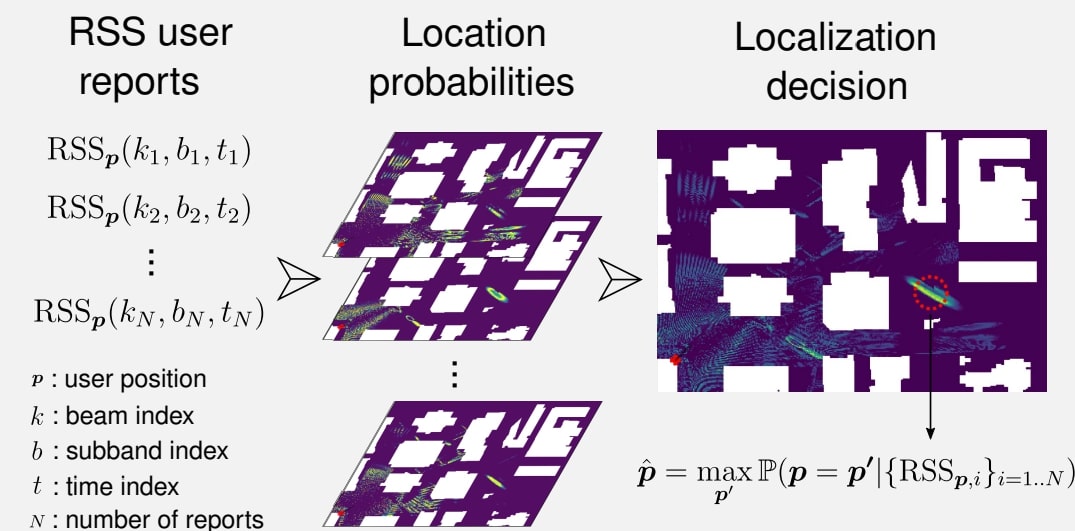
Bibtex
@misc{morais2024localizationdigitaltwinmimo,
title={Localization in Digital Twin MIMO Networks: A Case for Massive Fingerprinting},
author={João Morais and Ahmed Alkhateeb},
year={2024},
eprint={2403.09614},
archivePrefix={arXiv},
primaryClass={cs.IT},
url={https://arxiv.org/abs/2403.09614},
}